Preventive Maintenance with RFID, RTLS, and IoT
​RFID allows precise identification and history tracking of assets, while RTLS ensures real-time localization of equipment for efficient task execution. IoT sensors provide real-time data on critical asset parameters (e.g., vibration, temperature, and energy consumption), enabling predictive alerts that allow organizations to transition from reactive to proactive maintenance. Organizations implementing these solutions on a unified platform gain significant operational advantages, such as extended asset lifespan, reduced maintenance costs, improved regulatory compliance, and enhanced productivity.
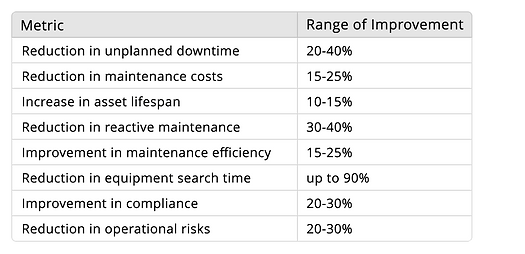
​Key Impacts and Metrics​
-
20-40% reduction in unplanned downtime through real-time monitoring and predictive maintenance alerts.
-
30-40% reduction in reactive maintenance by shifting to proactive, condition-based interventions.
-
20-25% reduction in maintenance costs through timely servicing and optimized resource allocation.
-
Up to 90% reduction in equipment search time using real-time location data from RTLS.
-
10-15% increase in asset lifespan, delaying costly capital replacements.
-
25-35% reduction in product spoilage by ensuring optimal equipment operating conditions in temperature-sensitive environments.
​ Key Components and Capabilities
​
-
Automated Asset Identification and Maintenance Records (RFID)
-
RFID tags attached to assets store unique identifiers and maintenance history, enabling automated updates of maintenance logs.
-
Maintenance personnel can quickly scan RFID tags to access maintenance schedules, history, and upcoming tasks.
-
Key Metrics:
-
Reduction in manual maintenance logging errors: up to 80%
-
Improvement in asset tracking accuracy: 95-98%
-
Increase in maintenance compliance: 10-20%
-
-
-
Real-Time Location of Critical Equipment (RTLS)
-
RTLS systems enable the precise tracking of mobile equipment, ensuring timely maintenance by identifying the exact location of assets across large facilities.
-
Geofencing with RTLS helps enforce maintenance zones, ensuring critical machinery is serviced only in designated areas.
-
Key Metrics:
-
Reduction in equipment search time: up to 90%
-
Improvement in maintenance efficiency: 15-25%
-
Reduction in missed maintenance tasks: 20-30%
-
-
-
Condition Monitoring and Predictive Maintenance (IoT Sensors)
-
IoT sensors continuously monitor key parameters such as vibration, temperature, pressure, and energy consumption, identifying early warning signs of potential failures.
-
Predictive analytics, powered by machine learning models, provide insights into when an asset is likely to fail, allowing proactive maintenance.
-
Key Metrics:
-
Reduction in unplanned downtime: 20-40%
-
Increase in asset lifespan: 10-15%
-
Reduction in maintenance costs: 15-25%
-
-
-
Unified Maintenance Platform and Predictive Insights
-
Data from RFID, RTLS, and IoT systems is consolidated on a unified platform, providing a single source of truth for maintenance planning and execution.
-
Advanced analytics generate predictive insights, enabling better resource allocation, maintenance scheduling, and cost control.
-
Key Metrics:
-
Reduction in reactive maintenance: 30-40%
-
Improvement in maintenance planning efficiency: 25-35%
-
Reduction in operational risks: 20-30%
-
-
Case Studies with Success Metrics
Case Study 1: Predictive Maintenance in Manufacturing
Challenge: A large automotive parts manufacturer faced frequent unplanned downtime due to equipment failures, resulting in delayed production and high repair costs.
Solution: IoT sensors were installed on critical machinery to monitor temperature and vibration, while RFID tags were attached to all equipment for tracking maintenance history. A unified platform provided real-time alerts and predictive maintenance schedules.
Results:
-
Reduction in unplanned downtime by 35%, improving overall production efficiency.
-
Maintenance costs reduced by 20% through proactive servicing.
-
Increase in equipment lifespan by 15%, lowering capital expenditure on new machinery.
Case Study 2: Condition-Based Monitoring in Energy & Utilities
Challenge: A utility company managing a large network of transformers and substations struggled with sudden equipment failures and long downtimes during repairs.
Solution: IoT sensors were deployed to monitor critical parameters such as load, temperature, and oil pressure in real-time. RTLS was used to track mobile maintenance units, ensuring faster response times.
Results:
-
Reduction in reactive maintenance tasks by 40%, improving service reliability.
-
Reduction in maintenance response time by 25%, minimizing downtime during critical failures.
-
Improvement in regulatory compliance by 20%, reducing the risk of fines.
Case Study 3: Real-Time Equipment Monitoring in Healthcare
Challenge: A hospital faced frequent failures of critical medical equipment, such as MRI machines and ventilators, due to inadequate maintenance scheduling. This resulted in increased patient wait times and high repair costs.
Solution: RFID tags were attached to all medical equipment to automate maintenance history tracking, and IoT sensors were installed to monitor equipment usage and performance. A centralized platform provided predictive maintenance alerts.
Results:
-
Unplanned equipment downtime reduced by 30%, enhancing patient care.
-
Maintenance compliance improved by 25%, ensuring timely servicing of all critical assets.
-
Repair costs reduced by 20%, resulting in significant cost savings.
Case Study 4: Geofenced Maintenance Zones in Aerospace
Challenge: An aerospace manufacturer needed to ensure that maintenance on high-value aircraft components was only performed in designated zones to comply with strict safety regulations.
Solution: RTLS was implemented to track the real-time location of assets, and geofencing was used to enforce maintenance zones. RFID tags recorded maintenance history, while IoT sensors monitored environmental conditions.
Results:
-
Compliance with safety regulations improved by 30%, reducing regulatory risks.
-
Reduction in operational risks by 20%, improving overall safety.
-
Maintenance efficiency increased by 25%, reducing turnaround time for aircraft servicing.
Case Study 5: Smart Maintenance in Oil & Gas Pipelines
Challenge: An oil and gas company faced frequent unplanned shutdowns due to corrosion and pressure anomalies in its pipeline network. Traditional manual inspections were time-consuming and prone to delays.
Solution: The company deployed IoT sensors along the pipeline to monitor real-time pressure, temperature, and corrosion levels. RFID tags were attached to key inspection points, and maintenance data was integrated into a centralized platform. RTLS was used to track mobile inspection units.
Results:
-
Reduction in unplanned shutdowns by 40%, ensuring continuous operations.
-
Maintenance costs decreased by 25% due to timely, condition-based interventions.
-
Inspection efficiency improved by 30%, reducing the time taken to inspect the entire network.
Case Study 6: Enhancing Maintenance in Cold Storage Warehouses
Challenge: A global logistics provider managing temperature-controlled warehouses experienced frequent equipment failures (refrigeration units, compressors) that led to spoilage of perishable goods.
Solution: IoT sensors were installed on refrigeration units to monitor temperature and energy consumption. RFID tags tracked maintenance history, while RTLS enabled precise tracking of mobile maintenance teams. Predictive analytics generated alerts for early signs of equipment failure.
Results:
-
Reduction in product spoilage by 35%, improving profitability.
-
Maintenance response time reduced by 25%, minimizing downtime.
-
Energy consumption decreased by 20%, leading to operational cost savings.
Case Study 7: Real-Time Condition Monitoring in Mining Operations
Challenge: A mining company faced frequent breakdowns of heavy machinery, including excavators and conveyors, resulting in operational delays and high repair costs.
Solution: The company implemented IoT sensors to continuously monitor vibration, temperature, and load on critical machinery. RFID tags were attached to equipment for automated maintenance tracking, and RTLS provided real-time location of mobile assets across vast mining sites.
Results:
-
Unplanned downtime reduced by 30%, improving overall productivity.
-
Maintenance compliance improved by 20%, ensuring timely servicing of critical assets.
-
Maintenance efficiency increased by 25%, reducing total operating costs.
Case Study 8: Predictive Fleet Maintenance in Transportation
Challenge: A logistics company managing a large fleet of delivery vehicles faced high repair costs and frequent vehicle breakdowns, leading to delayed deliveries and reduced customer satisfaction.
Solution: IoT sensors were installed on vehicles to monitor key parameters, including engine temperature, oil pressure, and brake wear. RFID tags tracked each vehicle’s maintenance history, while a unified platform generated predictive maintenance alerts based on sensor data.
Results:
-
Breakdown incidents reduced by 40%, improving fleet availability.
-
Maintenance costs reduced by 20%, lowering operational expenses.
-
Delivery accuracy improved by 15%, enhancing customer satisfaction.
Case Study 9: Predictive Maintenance in Heavy Construction Equipment
Challenge: A large construction company experienced frequent breakdowns of heavy machinery (e.g., excavators, cranes, bulldozers) due to delayed maintenance, leading to costly project delays and reduced operational efficiency.
Solution: IoT sensors were installed on all heavy equipment to monitor engine health, hydraulic pressure, and operating temperature in real-time. RFID tags were attached to track maintenance history, and RTLS was deployed on large construction sites to ensure rapid location of machinery for servicing. A centralized platform consolidated all data, enabling predictive maintenance alerts.
Results:
-
Reduction in unplanned downtime by 40%, ensuring uninterrupted project execution.
-
Maintenance costs reduced by 20% due to timely servicing.
-
Asset utilization improved by 15%, increasing overall productivity.
-
Repair time reduced by 25%, minimizing delays.
Case Study 10: Condition-Based Monitoring for Cranes in Construction
Challenge: A crane operator company faced frequent mechanical failures of tower and mobile cranes, resulting in operational risks and increased maintenance expenses.
Solution: IoT sensors were deployed on cranes to monitor structural integrity (e.g., load stress, vibration) and operating conditions. RFID tags logged maintenance history, and RTLS provided precise geolocation of cranes across multiple construction sites. Predictive maintenance models were developed based on historical data.
Results:
-
Reduction in crane failure incidents by 30%, improving safety.
-
Maintenance efficiency increased by 25%, lowering total operating costs.
-
Regulatory compliance improved by 20%, ensuring adherence to safety standards.
Case Study 11: Preventive Maintenance in Utility Substations
Challenge: A utility provider managing hundreds of substations faced frequent transformer failures and long downtimes due to delayed maintenance, resulting in service disruptions and customer dissatisfaction.
Solution: IoT sensors were installed on transformers to monitor temperature, oil levels, and load conditions in real-time. RFID tags were attached to all critical components for easy identification, and RTLS tracked mobile maintenance teams to improve response times. A predictive maintenance platform analyzed sensor data to generate alerts before failures occurred.
Results:
-
Unplanned downtime reduced by 35%, ensuring reliable power supply.
-
Maintenance costs reduced by 20%, minimizing repair expenses.
-
Maintenance response time improved by 30%, reducing outage duration.
-
Asset lifespan increased by 10%, delaying the need for capital-intensive replacements.
Case Study 12: Smart Maintenance of Water Treatment Plants
Challenge: A water utility company experienced frequent equipment failures in its treatment plants, including pumps, compressors, and valves, leading to high repair costs and water supply disruptions.
Solution: IoT sensors were installed to monitor flow rates, pressure, and energy consumption of critical equipment. RFID tags tracked the maintenance history of all plant components, while RTLS was used to track mobile maintenance staff across large treatment plants. A centralized dashboard provided predictive alerts and automated maintenance scheduling.
Results:
-
Reduction in reactive maintenance by 40%, leading to better resource allocation.
-
Energy consumption reduced by 20%, lowering operational costs.
-
Equipment downtime reduced by 30%, ensuring consistent water supply.
-
Regulatory compliance improved by 25%, reducing penalties and fines.
Case Study 13: Real-Time Monitoring in Wind Farms
Challenge: A renewable energy company managing multiple wind farms faced challenges in maintaining turbines due to unpredictable failures, resulting in downtime and revenue losses.
Solution: IoT sensors were deployed on wind turbines to monitor vibration, rotor speed, and power output. RFID tags were used to maintain a log of each turbine’s servicing history, while RTLS tracked maintenance teams across remote wind farm locations. Predictive models were applied to forecast failures based on sensor data.
Results:
-
Reduction in turbine downtime by 30%, improving energy generation.
-
Maintenance costs reduced by 20%, increasing profitability.
-
Maintenance response time improved by 25%, ensuring quicker issue resolution.
-
Increase in turbine lifespan by 15%, reducing long-term capital expenditure
Case Study 14: Maintenance Optimization for Underground Utility Networks
Challenge: A city’s municipal utility department struggled with maintaining underground networks (gas, water, and sewage pipelines) due to the high cost and difficulty of inspections, leading to occasional service disruptions and safety hazards.
Solution: IoT-enabled sensors were installed at critical nodes to monitor pressure, flow rates, and gas leaks. RFID tags were deployed at key junctions to log inspection data, and RTLS tracked maintenance vehicles to optimize dispatching. A unified platform provided real-time alerts for anomalies, enabling proactive repairs.
Results:
-
Service disruptions reduced by 30%, improving public satisfaction.
-
Inspection time reduced by 25%, lowering operational costs.
-
Maintenance compliance improved by 20%, reducing regulatory risks.
-
Safety incidents reduced by 15%, enhancing operational safety.
Key Impacts and Consolidated Metrics
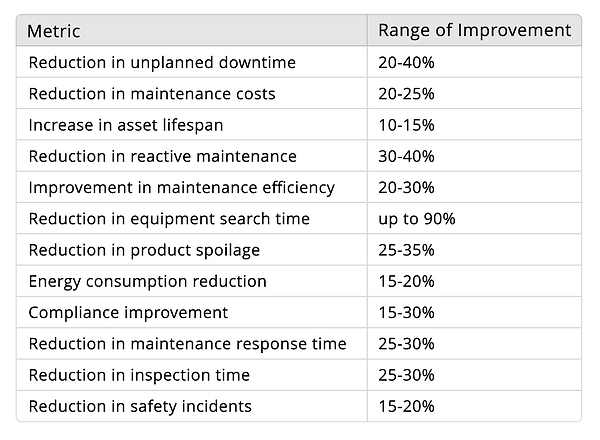